As technology continues to advance, the importance of data and its analysis has become crucial for businesses and organizations around the world. With the rise of artificial intelligence (AI) and machine learning (ML), there is a growing demand for professionals who can develop and deploy ML models that can help automate processes, make predictions, and solve complex problems.
In this article, we will explore how you can master machine learning with the help of Amazon Web Services (AWS). We will cover everything from understanding the basics of machine learning to learning how to use AWS tools and services for ML development and deployment. So let’s dive in and discover the world of machine learning with AWS.
Introduction to Machine Learning with AWS
Before we delve into the specifics of mastering ML with AWS, let’s first understand the basics of machine learning. In simple terms, machine learning is a subset of AI that enables computers to learn and make decisions without being explicitly programmed. It involves using algorithms and statistical models to analyze large datasets and find patterns that can be used to make predictions or take actions.
Now, let’s see how AWS fits into the picture. AWS is a cloud computing platform that offers a wide range of services, including storage, networking, analytics, and machine learning. By leveraging AWS, you can access powerful ML tools and services that can help you build, train, and deploy ML models quickly and easily.
Understanding the Foundations of Machine Learning
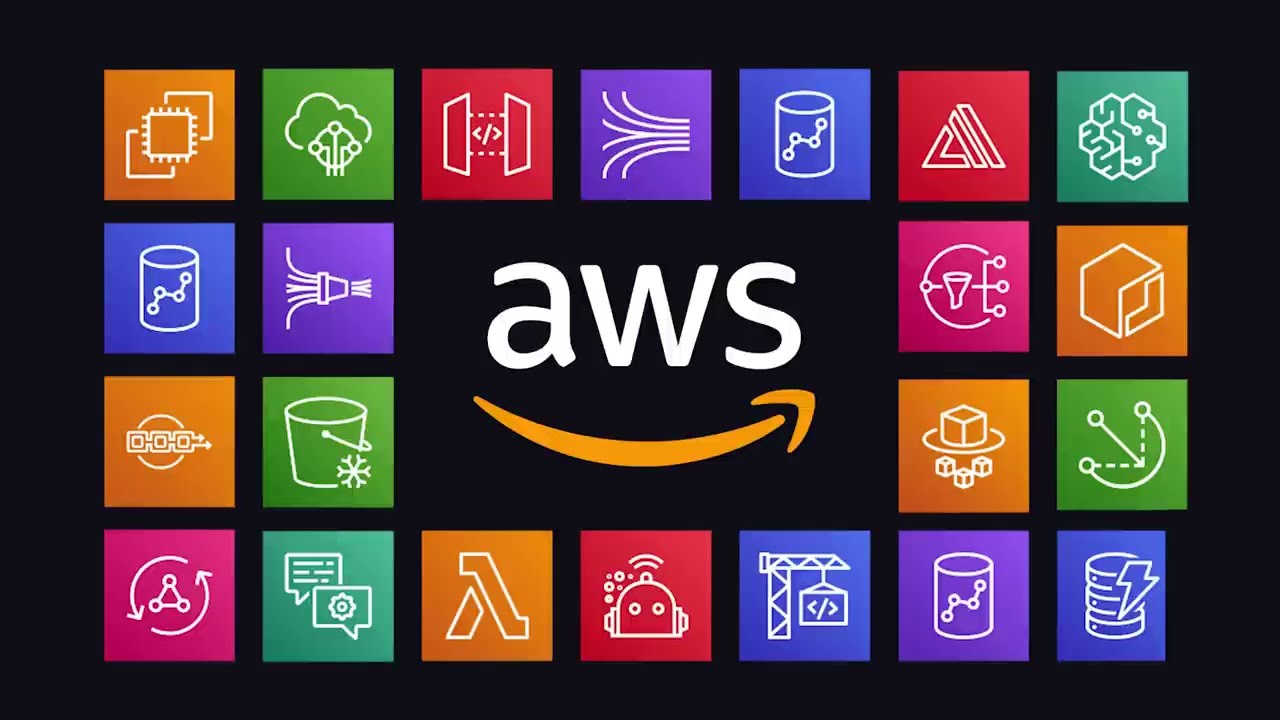
To master machine learning with AWS, it is essential to have a strong foundation in the principles and concepts of machine learning. Let’s explore some key topics that you should familiarize yourself with before moving on to AWS-specific tools and services.
1. Types of Machine Learning
There are three main types of machine learning: supervised learning, unsupervised learning, and reinforcement learning. Understanding these types is crucial as they determine the kind of data you need, the algorithm to use, and the type of problem you can solve.
- Supervised Learning: This type of ML involves training a model on labeled data, where the desired outcome is known. The model then uses this knowledge to make predictions on new data.
- Unsupervised Learning: In contrast to supervised learning, here, the data is unlabeled, and the model must identify patterns and relationships without any guidance.
- Reinforcement Learning: This type of ML involves training a model to take actions in an environment to maximize a reward or minimize a penalty.
2. Data Preprocessing
Data preprocessing is an essential step in ML as it involves cleaning and transforming data before feeding it to an algorithm. This is necessary to ensure that the data is in a format that can be used by the model effectively. Some common techniques for data preprocessing include handling missing values, normalization, and dimensionality reduction.
3. Evaluation Metrics
Evaluation metrics are used to measure the performance of a trained ML model. These metrics can vary depending on the type of problem and the type of data being used. Some commonly used evaluation metrics include accuracy, precision, recall, and F1 score.
Mastering Machine Learning with AWS Tools and Services
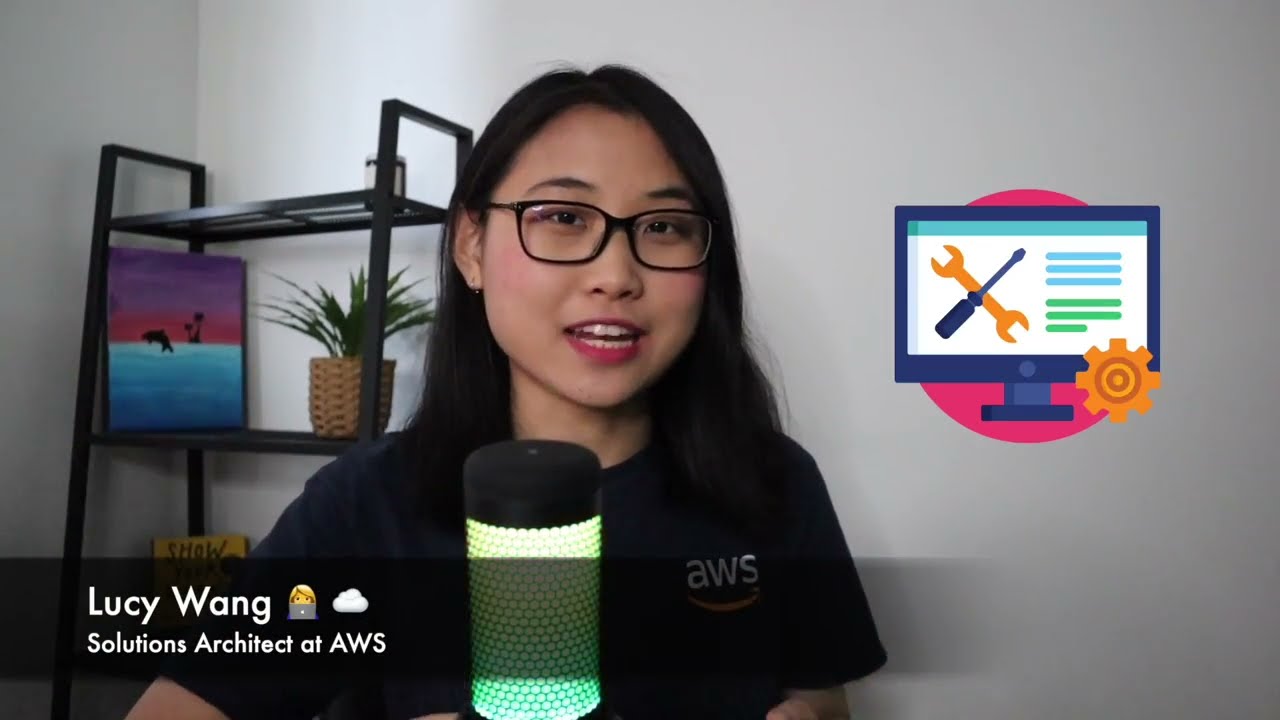
Now that we have covered the basics of machine learning let’s explore how you can use AWS to develop and deploy ML models.
1. Amazon SageMaker
Amazon SageMaker is a fully managed ML service that enables developers and data scientists to quickly build, train, and deploy ML models at scale. It provides a platform for all stages of the ML development process, from data exploration and preprocessing to model training and deployment.
How to Use Amazon SageMaker
To use Amazon SageMaker, you first need to create a notebook instance, which is a cloud-based Jupyter notebook that allows you to write and run code. You can then import your dataset and use SageMaker’s built-in algorithms or bring your own custom code to train and deploy your model.
Example
Let’s say you want to build a sentiment analysis model using Amazon SageMaker. You can use the built-in linear learner algorithm to train your model on a dataset of customer reviews. Once the model is trained, you can then deploy it to an endpoint, where it can receive new data and make predictions in real-time.
Comparison with Other Services
Compared to other ML tools and services, Amazon SageMaker offers a more integrated and streamlined approach to building and deploying models. It also provides the ability to customize and bring your own algorithms, making it suitable for advanced users.
Advice for Beginners
For beginners, it is recommended to start with Amazon SageMaker as it provides a user-friendly interface and takes care of the heavy lifting involved in setting up ML infrastructure. Additionally, there are plenty of tutorials and resources available to help you get started.
2. Amazon Rekognition
Amazon Rekognition is a deep learning-based image and video analysis service that can be used for a variety of applications such as facial recognition, object detection, and scene understanding. It makes it easy for developers to add image and video analysis capabilities to their applications without needing any machine learning expertise.
How to Use Amazon Rekognition
To use Amazon Rekognition, you simply need to upload images or videos to the service and specify the type of analysis you want to perform. The service then returns a response with the results, which can be integrated into your application.
Example
Let’s take the example of a retail store that wants to enhance its security measures. By using Amazon Rekognition, the store can set up a system that can detect and alert employees if someone is wearing a mask or carrying a weapon.
Comparison with Other Services
Compared to other image and video analysis services, Amazon Rekognition offers a more comprehensive set of features and can handle a wide range of use cases. It also integrates seamlessly with other AWS services, making it a go-to choice for businesses using AWS.
Advice for Beginners
For beginners, it is recommended to try out the demos and sample applications provided by Amazon Rekognition before diving into building your own models. This will help you understand how the service works and give you ideas for incorporating it into your own projects.
FAQs
Q: Can I use AWS ML services without any prior experience in machine learning?
A: Yes, AWS provides tools and services that are suitable for users with varying levels of expertise. You can start with the basics and gradually move on to more advanced services as you gain more experience.
Q: Do I need to have coding skills to use AWS for machine learning?
A: While having coding skills can be helpful, it is not a requirement for using AWS for machine learning. Many of the services have user-friendly interfaces that allow you to build and deploy models without writing a single line of code.
Q: Is AWS suitable for large-scale ML projects?
A: Absolutely. AWS offers scalable and fully managed services that can handle large datasets and complex ML models. With AWS, you can easily train and deploy models at scale, making it an ideal platform for large-scale ML projects.
Q: Are there any free resources available for learning machine learning with AWS?
A: Yes, AWS provides a variety of free resources, including tutorials, documentation, and training courses, to help users learn how to use their ML services.
Q: Can I use AWS for real-time predictions?
A: Yes, many of the ML services offered by AWS can be used to make real-time predictions, such as Amazon SageMaker and Amazon Rekognition.
Conclusion
In this article, we have covered the fundamentals of mastering machine learning with AWS. We have explored key topics related to machine learning, such as types of ML, data preprocessing, and evaluation metrics. We have also looked at two popular AWS services, Amazon SageMaker and Amazon Rekognition, and how you can use them for your machine learning projects.
Whether you are a beginner looking to get started with machine learning or an experienced data scientist looking to leverage the power of the cloud, AWS has got you covered. With its wide range of tools and services, you can easily build and deploy powerful ML models and take your projects to the next level. So start exploring and mastering machine learning with AWS today!